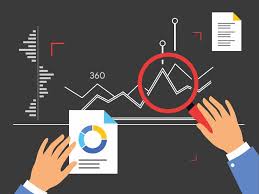
Order Instructions:
This is a forecasting assignment for Business Analysis and Modelling MBA module. The core text used Quantitative Analysis for Modelling by Render and Stair.
This forms part of a group assignment.
Further details will be sent across attached later.
SAMPLE ANSWER
Forecasting Assignment
Forecasting is widely regarded as a method of predicting events that may happen in the future. The accepted definition of forecasting is the process of generating predictions concerning the future by analyzing trends emanating from past and present data (Hirschmann, 2008). Data from forecasts is considered to be estimation since the future is unpredictable, but it is a reliable source of information for planning purposes owing to the future projections of the firm. Due to the unpredictability, forecasting methods provide an allowance for risk and uncertainty, and such a degree of uncertainty is usually indicated succeeding the forecast (Hirschmann, 2008). On a wider scope, however, forecasts are imperative by enhancing the quality of decisions due to risk reduction. Forecasting relies on the statistical discipline by applying models such as time series analysis and relies on data generated from vertical and horizontal analysis (Hirschmann, 2008).
Vertical analysis is the assessment of data amongst different organizations in the same industry, and in most cases organizations of similar sizes (Hirschmann, 2008). This is also called longitudinal analysis. Horizontal analysis is the examination of data for an organization over a period, for instance the past ten years. It can also be referred to as cross-sectional analysis (Hirschmann, 2008). Forecasting methods can be categorized into quantitative and qualitative methods. The quantitative methods predict future data by the assumption that the data is function of past data. The model utilizes past numerical data to develop trends that can be extrapolated to predict future data (Hirschmann, 2008). Exponential smoothing, linear predictions and moving averages are some of the quantitative methods of forecasting. Unlike quantitative forecasting which relies on speculative data, qualitative forecasting is quite subjective (Hirschmann, 2008). The latter predicts the future by relying on judgements and opinions, for example of experts. This method is mostly used in instances where past data is not available. Examples of such models are the historical life cycle analogy and market research (Hirschmann, 2008).
Due to the advent of technology, there are several software that have been developed that are able to predict future outcomes. Amongst such include excel QM and QM for windows that are used in forecasying. Excel QM is one of the applications developed by Microsoft corporation and it is mainly used in mathematical and statistical computations. QM for windows is also an application by microsoft, with a centre focus on statistics (Hirschmann, 2008). Such applications are vital in easening the forecasting work, and provide a great deal of accuracy compared to the traditional methods such as regression analysis and use of coefficients such as the Pearson’s coefficient (Hirschmann, 2008). Such software are capable of determining variants such as coeeficients of correlation and coefficients of determination.
In this milieu, focus will be on the quantitative methods to predict future data, specifically the method of moving averages. The moving average method, also referred to as the running average, is among the several time series methods that utilize historical data in the estimation of future outcomes. This method analyzes data points through generating a succession of averages that are derived from a series of values or various segments that are successive (Chiarella, He & Hommes, 2005). Periodical data such as monthly sales may have random fluctuation every month despite a general trend being evident. Moving average helps in smoothing away these random changes (Chiarella, He & Hommes, 2005).
A moving average is the forecast for a period that takes the average of the previous periods (Chiarella, He & Hommes, 2005).The moving average takes the average of previous series collected from a preliminary fixed subset. This subset is shifted forward, hence creating a new subset of numbers. The average of this new subset is determined, and the process is replicated on the remaining data in the series (Chiarella, He & Hommes, 2005). The moving average results to the smoothing of fluctuations that are short-term and in the process underlining the long-term trends from the given data.
Among the functions of the moving average method is the identification of reversals and trends of data over a period. The method is also imperative in the determination of the levels of support and resistance (Chiarella, He & Hommes, 2005). In stock trading, the moving average is key in imparting vital trading signals, particularly by the identification of uptrends and downtrends. The moving averages are considered to be lagging indicators, per se, since they only confirm trends but do not predict new ones. There are two moving average methods that are commonly used; the simple moving average and the exponential moving average (Chiarella, He & Hommes, 2005). The simple moving average is the simple average of data such as securities spanning a number of time periods. The exponential moving average, on the converse, puts much focus on recent data compared to data relating to a past time period (Chiarella, He & Hommes, 2005).
The moving average has various characteristics. Foremost, the more the number of periods in the moving average, the greater the smoothing effect (Appel, 2012). Different moving averages produce different forecasts. Another charectiristic is that the more the randomness of data with underlying trend being constant then the more the periods should be involved in the moving averages (Appel, 2012). Moving averages, however, have various limitations. They depict equal weighing with disregard to how more recent data is more relevant. Moving average ignores data outside the period of the average thus it doesn’t fully utilise available data.Also, where there is an underlying seasonal variation, forecasting with unadjusted moving average can be misleading (Appel, 2012).
Exponential smoothing is a method that involves automatic weighing of past data with weights that decrease exponentially with time (Hyndman, 2008). Exponential smoothing has certain characteristics. Amongst them is that more weight is given to the most recent data (Hyndman, 2008). Secondly, all past data are incorporated unlike in moving averages. Less data is needed to be stored unlike in periodic moving averages (Hyndman, 2008).
Both the methods rely on time series. Time series, however, possesses various characteristics. They have a long term trend, and the tendency of the whole series is to rise and fall (Chiarella, He & Hommes, 2005). They also posess seasonal variations, implying short term periodic fluctuations in values (Chiarella, He & Hommes, 2005). Time series have cyclical variations, and these are medium term changes caused by factors which apply for a while then disappear, and come back again in a repetitive cycle (Chiarella, He & Hommes, 2005). Cyclic variations have a longer term than seasonal variations, for instance seasonal variations may occur once every year while cyclic variation occurs once every several years. Time series also have random residual variations, whereby these are non-recurring random variations for instance war, fire or a political coup (Chiarella, He & Hommes, 2005).
Differences between actual results and predictions may arise from many reasons. They may arise from random influences, normal sampling errors, choice of the wrong forecasting system or alpha value or simply that the future conditions turn out to be radically different from the past (McAuliffe, 2011). Whatever the cause, persons involved with forecating wish to know the extent of the forecast errors and various methods exist to calculate these errors (McAuliffe, 2011).
A commonly used technique, appropriate to time series, is to calculate the mean squared error of the deviations between forecast and actual values then choose the forecasting system or parameters which gives the lowest value of mean squared errors, such as akin to the least squares method of establishing a regression line (McAuliffe, 2011). Moving averages tend to be used for short to medium term forecasting (McAuliffe, 2011). Longer term forecasting is usually less detailed and is normally concerned with forecasting the main trends on a year to year basis. Other techniques could be used depending on the assumptions about linearity or non- linearity, and the number of independent variables. The least squares regression approach is often used for trend forecasting (McAuliffe, 2011).
References
Appel, G. (2012). The moving average convergence-divergence trading method: Advanced method. Toronto: Scientific Investment Systems.
Chiarella, C., He, T., & Hommes, C. (2005). A dynamic analysis of moving average rules. Amsterdam: Tinbergen Institute.
Hirschmann, K. (2008). Forecasting. Edina, Minn: ABDO Publishers.
Hyndman, R. J. (2008). Forecasting with exponential smoothing: The state space approach. Berlin: Springer.
McAuliffe, B. (2011). Forecasting. Mankato, MN: Creative Education.
We can write this or a similar paper for you! Simply fill the order form!